Annotation in Machine Learning: Revolutionizing Home Services with Keymakr
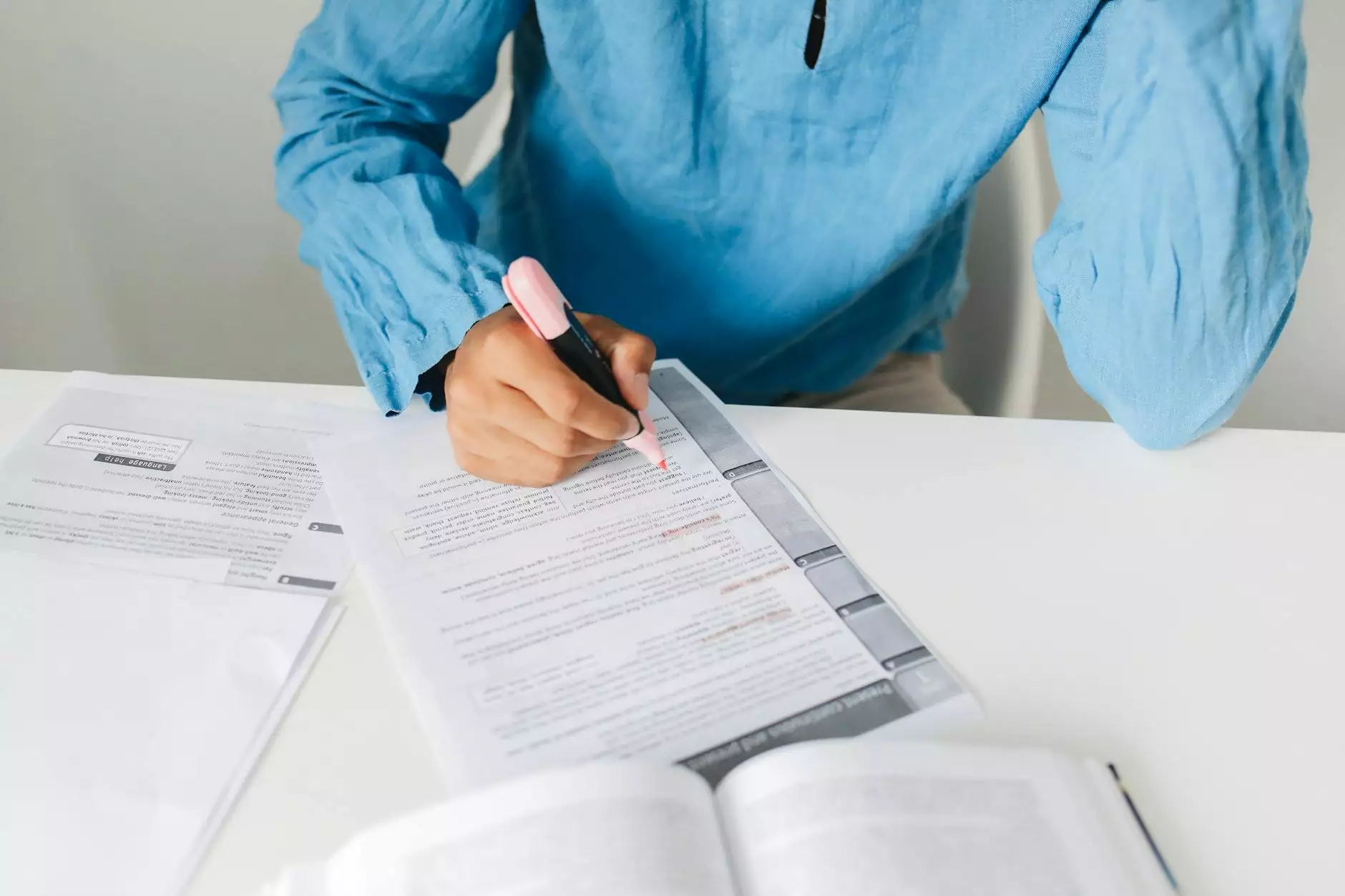
In the rapidly evolving landscape of technology, machine learning has emerged as a pivotal force driving innovation across various sectors. One of the fundamental aspects of machine learning is annotation, a process that plays a crucial role in training algorithms to recognize patterns, make informed decisions, and ultimately deliver superior services. In this article, we delve deep into the significance of annotation in machine learning and how it can elevate the standards of home services, particularly in the realms of keys and locksmiths offered by Keymakr.
What is Annotation in Machine Learning?
Annotation refers to the process of labeling data to make it interpretable for machine learning models. This involves identifying and tagging relevant objects or features within a dataset, providing the machine learning algorithms with the context they need to understand and learn from the data. In practical terms, this could mean annotating images, text, or even audio clips, allowing models to train effectively on a wide range of tasks.
Types of Annotation
- Image Annotation: Involves tagging images to highlight specific objects, boundaries, or characteristics.
- Text Annotation: Encompasses labeling text data, such as classifying sentiment or identifying named entities.
- Audio Annotation: Involves marking up audio files, useful in speech recognition and transcription services.
- Video Annotation: Includes tagging video content to assist in object tracking or event recognition.
The Importance of Annotation in Machine Learning
Annotation serves as the backbone of any successful machine learning project. Here are several reasons why it's essential:
1. Enhancing Model Accuracy
High-quality annotated data allows machine learning models to achieve greater levels of accuracy. By providing clear labels, models can better discern between different classes, leading to improved performance in tasks such as image recognition or natural language processing.
2. Facilitating Supervised Learning
Supervised learning techniques require labeled datasets to train models effectively. Annotation enables this process, helping algorithms learn the relationships between input data and desired outcomes.
3. Enabling Real-World Applications
Many real-world applications depend on precise data annotation. For instance, in home services, accurately identifying lock types or key specifications through annotated images can streamline the locksmith's service delivery.
How Annotation Benefits Home Services with Keymakr
In the home services industry, particularly for businesses like Keymakr that provide key and locksmith services, leveraging annotation in machine learning can lead to significant advantages:
1. Improved Service Delivery
By utilizing annotated datasets, Keymakr can develop machine learning models that enhance service delivery. For instance, an annotated database of various lock types and their mechanisms can help locksmiths identify issues quickly and accurately, leading to faster resolution times for customers.
2. Enhanced Customer Experience
Through the insights derived from machine learning, Keymakr can tailor its offerings to better meet the needs of customers. For example, understanding common lock problems in specific locales through data analysis can guide inventory management and service strategies.
3. Predictive Analysis for Home Security
Applying machine learning to annotated datasets can help Keymakr predict trends in home security needs. Models trained on customer feedback and service requests can provide insights into emerging security concerns in specific neighborhoods, allowing proactive adjustments to service offerings.
Challenges of Annotation in Machine Learning
While annotation is critical, it also comes with its set of challenges:
1. Time and Cost-Intensive
The process of annotating data can be both time-consuming and costly, especially when dealing with large datasets. Organizations must weigh these costs against the potential benefits.
2. Subjectivity and Inconsistency
Human annotators may introduce subjective views, leading to inconsistencies in the labeling process. This can affect the quality of the training data, ultimately impacting model performance.
3. Scalability Issues
As businesses grow, the need for annotated data can increase exponentially. Developing scalable annotation processes without compromising quality is a significant challenge for many organizations.
Best Practices for Annotation in Machine Learning Projects
1. Clear Guidelines for Annotators
Providing clear and detailed instructions for annotators helps ensure consistency and accuracy in the annotation process.
2. Utilize Automation Where Possible
Incorporating automated tools for annotation can significantly speed up the process and reduce costs. However, human oversight is still necessary to maintain quality.
3. Regular Quality Checks
Implementing regular quality control measures can help identify and rectify errors in the annotation process, ensuring high-quality training data.
4. Collaboration and Feedback
Encouraging collaboration among annotators and soliciting feedback can lead to improvements in the annotation process and data quality.
Future Trends in Annotation and Machine Learning
The field of annotation in machine learning is rapidly evolving. Here are a few trends to watch for:
1. Increased Use of AI in Annotation
Artificial intelligence systems are increasingly being used to automate the annotation process. This holds the potential to significantly reduce time and labor costs while improving accuracy.
2. Crowdsourcing Annotation Tasks
Crowdsourcing has become a popular method for gathering large quantities of annotated data quickly. Platforms enabling the crowd to participate in data annotation tasks are becoming increasingly common.
3. Developing Standardized Protocols
As the field advances, there is a growing need for standardized protocols that can guide the annotation process across various industries, including home services.
Conclusion: The Role of Keymakr in Embracing Machine Learning Annotations
In conclusion, annotation in machine learning is a vital component that significantly enhances the capabilities of businesses, especially in the home services sector. For a company like Keymakr, harnessing the power of machine learning annotations can lead to improved service delivery, enhanced customer experiences, and effective solutions to home security challenges. By staying abreast of trends and adopting best practices in data annotation, Keymakr is well-positioned to lead in the evolving landscape of home services.
By understanding and implementing the concepts of machine learning annotations, Keymakr can not only streamline its operations but also set a new standard for excellence in the locksmith and home services industry.